Story
Spotted! Floating Plastic Litter Detected and Categorised Using Artificial Intelligence (AI)
20 July 2022
A new AI technique pioneered by scientists at PML uses images from a vessel-mounted camera to identify different types of marine plastic.
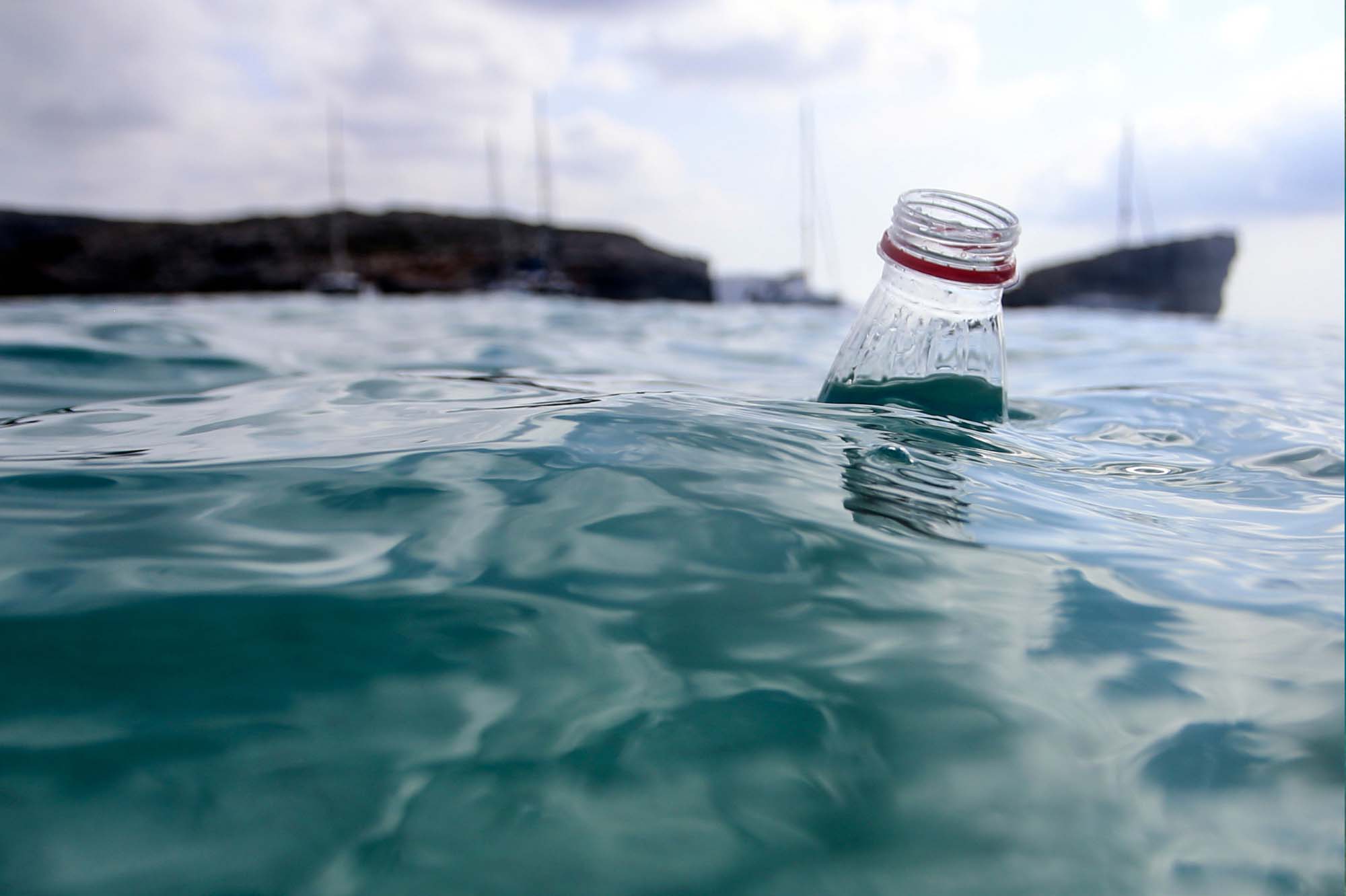
Plastic waste is a major part of the global pollution crisis, affecting marine organisms and ecosystems and, in turn, posing a threat to human health. To support efforts to mitigate the issue it is vital that marine plastic can be monitored effectively, however this is challenging given the scale, complexity and time required to do so manually.
As such, a team of scientists from Plymouth Marine Laboratory have “trained’ an Artificial Intelligence (AI) model to recognise and classify the different types of marine plastic captured in images shot by a video camera mounted on the side of a boat.
Funded by the PML internal research program and the European Space Agency (ESA), the innovative study – titled “Detection and Classification of Floating Plastic Litter Using a Vessel-Mounted Video Camera and Deep Learning” – was carried out as part of an undergraduate placement project, with the results now published in the journal Remote Sensing.
The AI model itself was trained using the MAGEO supercomputer (Massive GPU Cluster for Earth Observation) which is based at PML and operated by the Natural Environment Research Council Earth Observation Data Acquisition and Analysis Service (NEODAAS).
The model was able to classify the presence or absence of plastic in an image with an accuracy of 95% and capable of differentiating different types of plastic – for example a plastic bag or bottle – with an accuracy of 68%.
It is now envisaged that the technique could be more widely applied using crewed or autonomous vessels, such as PML’s proposed long-range autonomous research vessel, the Oceanus, thereby revolutionising existing capabilities to monitor floating plastic litter.
Dr Victor Martinez Vicente, Senior Scientist at PML said:
“In situ harmonised and simplified observations of floating marine plastic debris are currently very limited in the literature. We have aimed to tackle the scarcity of these observations through our research on low-cost automated observations. We hope that this initial step will lead to an increase of in situ observations everywhere, but especially in poorer countries where marine litter is usually a great problem.
With the increase of these observations, we expect to support the validation of algorithms from current sensors and the development of future satellite missions. Properly validated satellite algorithms will allow us to use remote sensing techniques to monitor the progress towards Sustainable Development Goals (in particular index SDG 14.1.1.b) at global scale.”
Sophie Armitage, the University of Exeter Undergraduate student who undertook the study as part of her placement at PML said:
“After my second year of University, I decided to take a placement year as I wanted to gain as much practical experience as possible and learn more about the industry in which I was aspiring to work in. After learning about the wide variety of innovative and fascinating projects ongoing at PML, I was enthusiastic to get involved.
During my year at PML, I have been giving numerous exciting opportunities to learn and advance my career. Specifically, this project pushed me out of my comfort zone and allowed me to learn many valuable new skills. From having a non-computing background and no previous knowledge of machine learning, I am very proud of this work as there was a very steep learning curve in which I had to overcome. Yet along the way I have found a new interest in remote sensing and machine learning – learning from the experts at PML – which I hope to continue in my future career, This work is very important to me as I am eager to assist in the battle to mitigate and prevent the devastating effects of marine plastic pollution on our ecosystems.”
Abstract: https://www.mdpi.com/2072-4292/14/14/3425
PDF Version: https://www.mdpi.com/2072-4292/14/14/3425/pdf
Related links:
https://www.pml.ac.uk/Science/Artificial-Intelligence-and-machine-learning
https://www.pml.ac.uk/Science/Plastic-pollution
https://www.pml.ac.uk/Science/Cleaner-seas
Figure 1. Summary of the method from collecting data on the PML Explorer to model training and plastic detection using the AI algorithm.